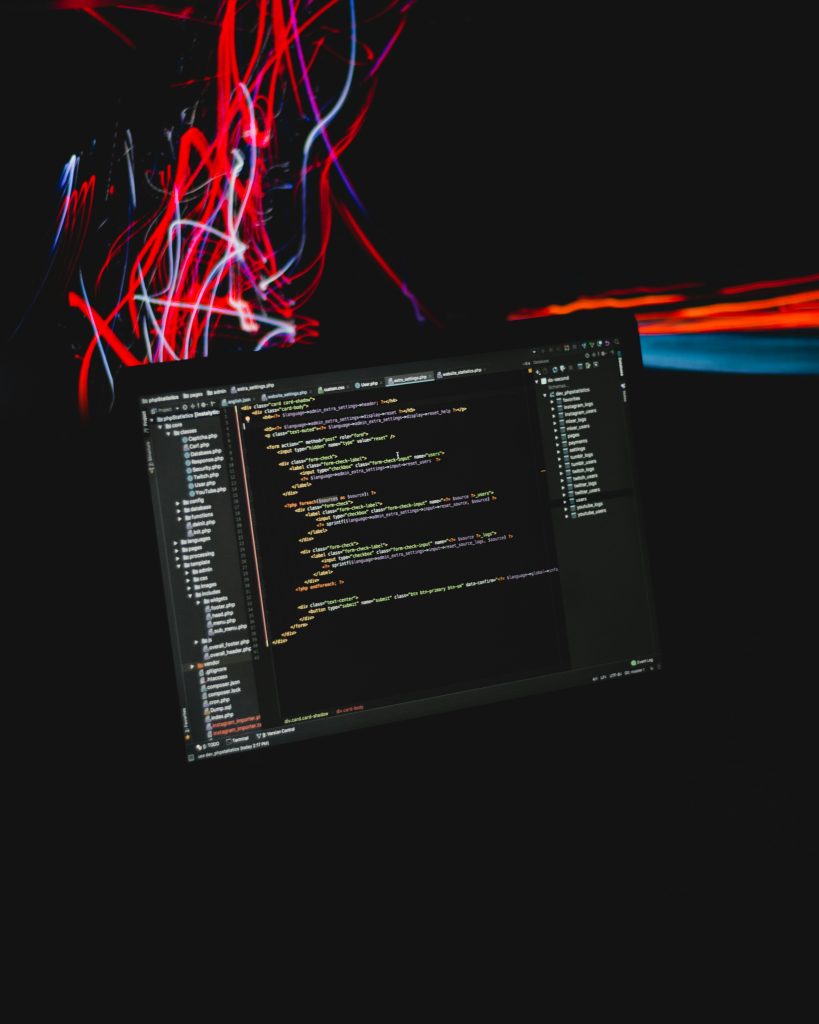
With all the daily hype it is probable that many people think that Generative AI is the only AI in town. And as Helen Beetham says with “growing scepticism from investors and push-back from almost every commercial sector against the hype around AI productivity”, as well as predictions over the cost of operations like Open AI, a wide range of ethical concerns including the amount of power and water Gen AI consumes and of course the ongoing issues around bias and ‘hallucinations’ , it is little wonder that many are beginning to see Generative AI and just another technological bubble.
In his Social Warming newsletter published today Charles Arthur says:
The problem with LLM is that people place too much trust in them. "They think ChatGPT is a search engine; it isn’t. They think it will give them factual help; it won’t. They think it will write essays; it sort of will, but the content will get worse rather than better over time" (this refers to a number of research projects (see for example, this report) showing that the quality of AI goes down - sometimes dramatically - as Large Language Models are trained using data that are at least partially generated by itself or other LLMs.
Charles Arthur goes on to clarify the difference between Generative AI and Machine Learning.
Finally, a word about machine learning—which I distinguish from generative AI because it tends to be focused on solving particular domain-specific problems, such as reading X-rays, or examining food on a production line, or helping people learn to play chess. For this, I think there’s a rosy future: the training is relatively easier (because the domain is limited) and the benefits easier to see. But it’s not where the attention is. In that sense, this is the perfect time for machine learning companies to jump in and take advantage. The whole space is open.
I wonder if this distinction is going to permeate the discussion over AI in Education in the coming months.